Don’t forget the rest of AI
- Jonathan Baier
- Dec 11, 2024
- 4 min read
How the hype for GenAI has blurred our understanding of “AI”
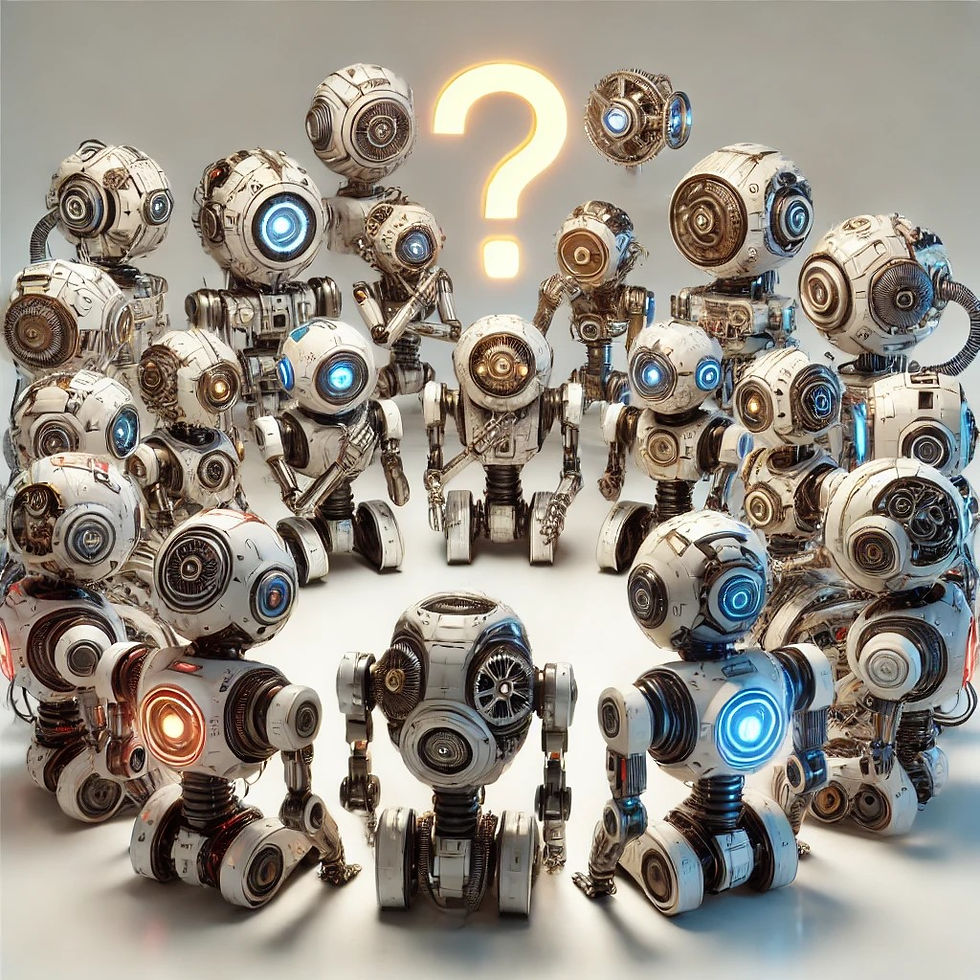
These days the AI label is everywhere, whether you are reading a technical publication, listening to a podcast (tech or not), or even just buying soda (seriously, look up Coca-Cola Y3000). However, the extent to which Artificial Intelligence is actually used varies significantly. Many stories even discuss how AI is reasoning or focus on the catastrophic outcomes from AI reaching sentience also labeled as Artificial General Intelligence (AGI). Regardless of the accuracy of such claims, a more nuanced confabulation is occurring.
Since the launch of ChatGPT in November 2022, Generative AI has taken hold of our collective consciousness to the point that it is easy to assume that any mention of AI is short for this kind of Generative AI or Large Language Model. To make matters worse there is broad misunderstanding of how “intelligent” even the state-of-the-art AI models have become.
To be clear, the misattribution of AGI and sentience is certainly a problem and risk for society, but I believe there is more immediate risk for businesses. Hidden beneath the avalanche of news is a tendency to simply paint all AI technology with the same brush or worse assume that if an organization is not using ChatGPT or GenAI in production then they don’t need an AI strategy or an update to their operational governance.
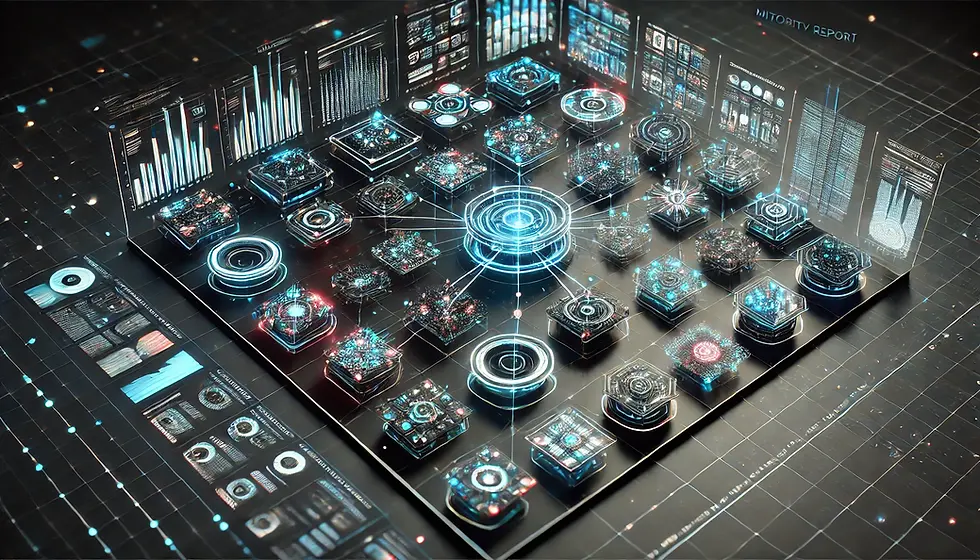
While Large Language Models, LLMs, have represented a big leap forward and stolen the stage as of late, there are likely many “AI” projects that already existed in your organization long before ChatGPT. In fact, several of these projects are likely already in use by production services.
Back when we were helping many enterprises to make the move to cloud computing we saw the more mature organizations adopt a more sophisticated data and analytic strategies as well. In the early 2010s this was often under the banner of “Big Data”, a term that has origins much earlier but reach a peak in the hype cycle around that time. This trend eventually led to Data Lake and Machine Learning initiatives as organizations matured their capabilities.
Fast forward to today where Generative AI is the latest buzz word. However, what might surprise some technology leaders who are not looking at Generative AI, or think it doesn’t apply to some of their projects, is that changes are occurring around the globe that will likely require changes to every organization’s IT department. While the news has focused on LLMs and ChatGPT, governments and regulators have felt the pressure to act to guide and, in some cases, regulate. But what many don’t realize is that many of these responsible AI frameworks were already under development for the traditional machine learning and artificial intelligence technologies before the world met ChatGPT.
While these frameworks were adapted to cover generative AI as well, many of the guidance documents and regulations include a broad definition of AI that can include simple regression and clustering techniques that have been common in ML efforts for close to a decade.
In fact, the EU AI Act defines AI as “A machine-based system designed to operate with varying levels of autonomy and that may exhibit adaptiveness after deployment and that, for explicit or implicit objectives, infers, from the input it receives, how to generate outputs such as predictions, content, recommendations, or decisions that can influence physical or virtual environments.”
By this broad definition one could argue that even certain statistical based functions qualify. While the EU may be one of the few areas to pass regulation into law, there is increasing guidance for responsible and ethical use of AI in practically every continent in the world (Though I am not aware of any guidance specific to Antarctica 😊).
In most cases the guidance emphasizes the need to create fair outcomes for their citizens whether focusing on biased models or intentional deep fakes. What again may be misunderstood is that an LLM or Generative AI is not needed to create a biased outcome. In fact, biased models are often the results of biased data and/or insufficient data governance.
It should also be noted that adversaries are not limiting their attacks to Generative AI either. While new AI enabled chat interfaces may attract attention, even the integrity and fairness of traditional ML based models can be impacted through data poisoning, supply chain attacks, or sensitive information exposures.
The takeaway is simple here: you shouldn’t wait to mature your data and AI governance or to establish a clear AI strategy and guidelines. The technical debt is already accruing, but the sooner you start the easier it will be to shape the direction and avoid costly adjustment further down the road.
Shameless plug, if you need help from folks who understand the nuance of new technologies and emerging regulations, we can help. Contact us to chat more and learn how we can help you modernize your data, AI, and cybersecurity programs.
Comments